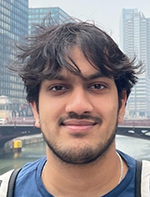
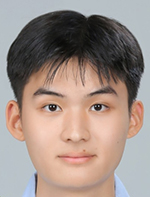
Two University of Illinois undergraduate students were finalists in the 2024 COVID Information Commons Student Paper Challenge. Arnav Aggarwal (Statistics & Mathematics) and Sangjun Ko (Statistics) received third place for their project “Identifying and Addressing the Socioeconomic and Mental Health Impacts of COVID-19 in Mexico: A Data-Driven Approach Using ENCOVID-19.” The COVID Information Commons (CIC) is an NSF-funded resource developed by Midwest Big Data Innovation Hub (MBDH) collaborators at the Northeast Big Data Innovation Hub.
We asked Sangjun and Arnav about their research for this project and their career plans.
Tell us about the project you completed as part of this challenge. What data did you use, and what approach did you select for analysis?
The project focused on analyzing the socioeconomic and mental health impacts of COVID-19 in Mexico, using the ENCOVID-19 dataset. This dataset captured various dimensions of household well-being during the pandemic, such as employment status, income fluctuations, mental health indicators, and demographic details like age, gender, and socioeconomic status. The goal was to identify vulnerable groups disproportionately affected by the pandemic and propose interventions to address these impacts in future crises.
We applied k-nearest neighbors (KNN) imputation for handling missing values and performed a geospatial analysis by integrating the survey data with a shapefile containing geographic boundaries of Mexican states. Our analysis revealed that young adults (18–35), females, and individuals from lower socioeconomic backgrounds were the most negatively affected, showing higher levels of anxiety, job loss, and income reductions.
What was interesting to you about this topic?
What intrigued us about this topic was how it explored the complex intersection between public health, mental health, and economics. This pandemic impacted every facet of life, but its effects were unevenly distributed, particularly among vulnerable populations. Therefore, investigating how different demographic and socioeconomic groups were affected allowed us to shed light on the inequalities that were exacerbated during this crisis.
What was surprising to you about what you learned from this project?
One surprising insight was the degree to which mental health and economic challenges were interconnected, especially among lower socioeconomic groups and females. Data analysis shows that while all socioeconomic groups faced challenges, even mid- to upper-socioeconomic levels experienced significant financial strain. However, these groups demonstrated greater resilience in terms of life satisfaction and mental health compared with those from lower levels. This was interesting, as it highlights the critical role of social support systems and emphasizes the importance of targeted mental health and economic interventions to build resilience in future crises.
How did you get interested in data science, and how does it relate to your degree programs?
Sangjun Ko: I first became interested in data science during the Spring 2023 semester at the University of Illinois at Urbana-Champaign (UIUC) while taking STAT107 with Professors Karle Flanagan and Wade Fagen-Ulmschneider. This course introduced me to the foundations of data science, but what truly captivated me were the labs and micro projects that focused on meaningful, real-world issues. These projects opened my eyes to a broader perspective of data science—one that goes beyond coding and data analysis to leveraging data for solving complex problems and answering impactful questions. This shift in perspective is what sparked my passion for using data to drive meaningful change. Currently, as a senior majoring in Statistics with minors in Mathematics and Data Science, my degree program has been closely aligned with data science. I’ve taken several courses that emphasize both the theoretical and practical aspects of data science.
Arnav Aggarwal: I actually started off as just a Math major with a Computer Science minor. It wasn’t until I took a Statistics class that I began to see how all the subjects: math, computer science, and statistics seamlessly blended together. That’s when I realized how much I enjoyed working with data and finding patterns. The combination of logic from math, coding from computer science, and real-world applications from statistics sparked my interest in data science. I love uncovering insights from data and seeing how those insights can drive decision-making, which is why I ultimately pursued a path that incorporates all these elements.
What career interests do you have after graduation?
Arnav: After graduation, I’m looking to pursue a master’s degree in financial engineering, with a strong interest in high-frequency trading (HFT). I’m fascinated by how mathematical and statistical models can be applied to make split-second trading decisions. The idea of using these models to analyze data in real time, and execute trades within milliseconds and sometimes nanoseconds even, is incredibly exciting to me.
Sangjun: After graduation, I am considering two potential career paths: pursuing graduate school in statistics to further my expertise in the field or entering the workforce as a statistical consultant. Both options would allow me to apply my knowledge in statistics to solve real-world problems and continue developing my skills in data science.
What would you suggest to other students who are new to data science but want to learn more?
Sangjun: For students who are new to data science and eager to learn more, I highly recommend Kaggle projects and lessons as a great starting point. Kaggle offers a variety of hands-on projects and tutorials that can help you build practical skills in data analysis and machine learning, even if you’re a beginner. It’s also a fantastic way to explore different datasets and see how data science is applied in various fields.
Additionally, participating in competitions like the CIC student paper challenge can give you valuable experience in tackling real-world problems and collaborating with others.
Arnav: If you’re new to data science and want to learn more, my advice would be to start by getting hands-on with real data as soon as possible. Whether it’s through class projects, online datasets, or internships, the key is to practice applying what you learn. Data science can feel overwhelming at first, but breaking it down, starting with foundational tools like Python or R and basic statistics, will help.
Also, don’t hesitate to explore different areas within data science, like machine learning, data visualization, or even niche fields like financial data science, because that can help you discover what you’re truly passionate about. Finally, stay curious and keep learning! There’s always something new to explore in this field.
About the Midwest Big Data Innovation Hub
The Midwest Big Data Innovation Hub is an NSF-funded partnership of the University of Illinois at Urbana-Champaign, Indiana University, Iowa State University, the University of Michigan, the University of Minnesota, and the University of North Dakota, and is focused on developing collaborations in the 12-state Midwest region. Learn more about the national NSF Big Data Hubs community.