Nitrogen reduction in the Upper Mississippi River Basin
By Katie Naum
As extreme climate events become more frequent, some of their impact is visible—like the derecho that tore through Iowa in August 2020, leaving a wake of destruction in its path. Other impacts—including nutrient pollution in water systems—are less understood. In what ways will climate change affect the world around us? How can we use data science to better understand and adapt to the impact of climate extremes?
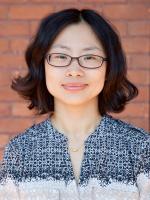
Chaoqun (Crystal) Lu is a quantitative ecosystem ecologist and assistant professor at Iowa State University, and a collaborator of the Midwest Big Data Innovation Hub. Her work focuses on water quality modeling, including the impact of extreme climate events and human activities on nutrient pollution. Her recent NSF CAREER award is titled “Understanding the dynamics and predictability of land-to-aquatic nitrogen loading under climate extremes by combining deep learning with process-based modeling”. The project will bridge the gaps between science and practice, sharing the most current knowledge of Earth system modeling to the public and making the complex concept of watershed management more concrete for the next generation of scientists, land managers, policy makers, and voters.
I spoke with Lu recently via Zoom to learn more about her work with water quality data. The following conversation has been edited and condensed for clarity.
Why is it important to study water quality here and now?
In the United States, nearly 60% of coastal rivers and bays have been degraded by nutrient pollution. Here in the Midwest, people have invested a lot of money and effort over the years to reduce nitrogen pollution. At the same time, climate-driven variations may far outweigh the effects of these nitrogen reduction practices. Increasing summer humidity, more frequent heavy rainfalls, and extreme floods have become a new normal in the central United States over the past few decades. There are a lot of unknowns about how extreme climate events have affected nitrogen leaching from soil and nitrogen loading through tiles, streams and rivers. Lots of data exist, though!
Policymakers need science-based management suggestions. As a researcher, I would like to benchmark my model with long-term measurements of water quality, and scale up from site-specific measurements to a broader region such as the Upper Mississippi River Basin. If we can figure out how to reduce nitrogen pollution here in the Midwest, the solution we come up with will be very likely to be effective elsewhere.
Can you tell readers more about the focus of your work, including your recent NSF CAREER award? (Congrats!)
I’m engaged in water quality modeling projects—studying, for example, the impact of nitrogen reduction practices on water quality. Our research team uses mathematical models to represent the physical processes involved in connected systems—the flow of water, the amount of nutrients used by plants or lost to runoff. We also quantify how climate change, land uses, and human management practices could affect nitrogen loading, and assess the effectiveness of nitrogen reduction practices in cleaning water.
The focus of this CAREER award is on how extreme climate events may affect nitrogen loading. My team wants to see how sensitive nitrogen leaching and loading are to events like these, which are increasing in the Midwest. We’re integrating machine learning approaches with a traditional process-based hydroecological model, using a large volume of water quality monitoring data that drains from various sized watersheds in the upper Mississippi–Ohio river basin. I want the key processes represented by traditional process-based models to be kept for water quality prediction, and at the same time improve the models’ outputs with “big data” and machine learning. Our integrated model uses data on water quality, weather, land cover, and human management practices, to better understand whether and where there are nitrogen pollution hotspots in the region.
What are some of the challenges in working with water data? What are the insights you hope to gain from your research?
One important challenge is just the enormous amount of variation in the data. If you look at a time series for hydrological flow, you see huge variation in the relationship between flow and nitrogen concentration. The challenge we have is to quantify how varied and why. Why do some small watersheds have larger variations than others? Why are some regions more sensitive to climate than others? Is this pattern we’re seeing caused by a specific event, or the legacy of many such events over time? We want to get the whole picture on nitrogen dynamics, from vegetation to soil to water to rivers, from small to large watersheds, at daily time steps, using modeling to recreate such processes.
In our work under this award, we’re planning to include more small watersheds and high frequency data sets. I’m looking forward to new insights from such data analysis. There is so much data over the past few decades to work with, and the technology of water quality monitoring has really improved.
How does deep learning contribute to watershed management?
Deep learning has been transformative for hydrological science and earth system science, yet few studies have used it to digest the big data of water quality monitoring. Meanwhile, high-frequency water quality monitoring data are increasingly available, especially in smaller watersheds and at shorter time scales. This brings new opportunities to test the relationship between flow and nitrogen concentration in response to climate extreme events. All of this motivates me.
Do you consider yourself a data scientist as well as an ecologist?
I consider myself an ecosystem ecologist, with data science skills. The question I want to find answers to are mostly ecological questions. Sustainability science, biogeochemical cycles, climate variability, natural and human drivers—these are all ecology questions. I say this even though I received training in ecosystem modeling and geospatial analysis for many years—but I consider these tools, the same way I consider machine learning a tool. I always keep my eyes open for tools that can help answer the ecological questions I care about. I tell my students this too: even if their degree or job title says ‘ecosystem modeler,’ I always hope they will step back and see the big picture.
How might interested stakeholders learn more or get involved?
We’ll be developing a project webpage where we will release research findings, future publications, and other relevant materials. Our results will be presented and disseminated to interested stakeholders through our collaborating institutions—not only to academic investigators, but also to the general public, because they are the people who actually make decisions on managing the land and improving the environment.
This is a very multidisciplinary project, and others may have different ways of thinking about and analyzing the problem that we haven’t considered. We would love to hear from other researchers interested in analyzing the problem from another angle. We are also working actively to seek collaborators and more grants to leverage this project, putting available data sources online to allow easy access.
What do you love most about your research?
Being a modeler is a very precious role. Through multi-scale modeling, we try to connect a lot of different people—field scientists, computational experts, engineers, economists, stakeholders, and policy makers—who can work together to understand and build a more sustainable world for us to live in. This provides a lot of opportunity to collaborate with people in different fields. As a quantitative ecosystem ecologist and ecosystem modeler, I can serve as a bridge between field scientists, extrapolating their findings, and decision makers, who want to see and understand ecological outcomes. The work is really useful and applicable in real life. I enjoy the endless possibilities and the feeling that my research is useful and applicable for our world.
Katie Naum writes on science & technology, climate change, and culture. Follow her @naumstrosity and read more at katienaum.com.
Get involved
Contact the Midwest Big Data Innovation Hub if you’re aware of other people or projects we should profile here, or to participate in any of our community-led Priority Areas.
The Midwest Big Data Innovation Hub is an NSF-funded partnership of the University of Illinois at Urbana-Champaign, Indiana University, Iowa State University, the University of Michigan, the University of Minnesota, and the University of North Dakota, and is focused on developing collaborations in the 12-state Midwest region. Learn more about the NSF Big Data Hubs community.