By Qining Wang
An expert in Eastern European Architecture, Professor Kimberly Zarecor tells us about her journey of building a highly interdisciplinary research team that takes data science into research on rural communities in Iowa.
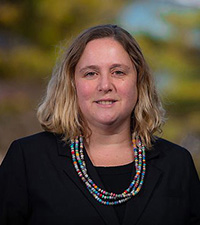
To some, architectural history and data science research may sound like oil and water—two fields that are almost impossible to mix well. However, Kimberly Zarecor, professor of Architecture at Iowa State University (ISU), leads her research team to create the perfect emulsion of many seemingly unrelated fields: sociology, statistics, industrial design, data science, architecture, and beyond.
With a research focus on small and shrinking communities in rural Iowa, not only does the team uncover the community efforts that keep some of these towns thriving, but the team is also offering the broader research community a valuable lesson on how to bring a wide range of expertise to projects and how experts from different fields can work together in harmony.
Zarecor found her inspiration to study Iowa’s shrinking towns from Ostrava, in the Czech Republic, a city she studied during her PhD research and later lived in for a semester as a Fulbright scholar. “[Ostrava] was part of a study in Europe called the Shrink Smart project, where [researchers] were looking at Ostrava as a shrinking post-industrial European city and questioned how to manage the governance of a relatively large city in the context of population loss.” As Zarecor shifted her primary research focus from architectural history in Eastern European cities to rural population loss in the Midwest, she realized the concept of shrinking smart could also be applied.
Zarecor and her collaborators started exploring the data-science component of shrinking smart with funding from a Smart & Connected Communities planning grant from the National Science Foundation (NSF) in 2017. Researchers at Iowa State University have been collecting data about the quality of life in small Iowa towns through the Iowa Small Town Poll since 1994, but “nobody had ever brought a data-science mindset to the analysis of [this] data.” The sociologists who had been collecting the data did not “think of [the poll] as a large set” and had not thought to build “a predictive model” from it.
Zarecor invited a computer scientist to be part of the planning grant team to transform the Small Town Poll data into training data, from which they could construct models to understand and predict the factors that influence people’s perceptions of quality of life in small rural communities. “We realized that what we were trying to understand is what are the actions that people in communities take as inputs into a system that results as outputs on the other side, as increases in perceptions of quality of life,” Zarecor explained. The planning grant team, consisting of a computer scientist, a sociologist, a community and regional planner, and two architects, found that “the best way to define [rural smart shrinkage] is that you are actively pursuing specific activities that you as a community can do together” that contribute to improved perceptions of quality of life even as population loss continues.
In 2020, Zarecor received another NSF grant of $1.5 million to continue this research and investigate strategies to address the data deficit in shrinking rural communities.
As the scope of the research expanded, so has Zarecor’s team. In addition to Zarecor and rural sociologist David Peters, who was also a Co-PI on the planning grant, the team now includes a community economic development specialist and a community arts specialist from ISU Extension and Outreach (both are also faculty in the College of Design at Iowa State), an industrial design faculty member, masters students from industrial design and community and regional planning, and for the data science work, three statistics faculty and three statistics PhD students. The Iowa League of Cities is also a partner on the project.
Coming to data science with little technical understanding, Zarecor approaches the data science component more from an intuitive rather than conceptual perspective: “It’s not that I understand the statistics, but I understand [the goals] as we go step by step . . . [and] the power of the tools that [the statisticians] are building.”
To lead such a highly interdisciplinary team, Zarecor thinks of herself as a bridge-builder within the team. Zarecor helps the members of her team understand data science by asking questions in a way that they can elicit responses that deepen the understandings of the nontechnical team members. “I like having that [bridge] function because it’s asking questions as a way of learning. For me, just the conversations with the data scientists helped me to better understand the data science part of our project.”
And the bridge function goes both ways. In addition to helping non-data-science experts learn more about the potential of data science, Zarecor also cultivates data scientists’ ability to contribute to projects that are community-based. “When it comes to community-based work, the assumption that this is not an expertise of its own is something that’s a challenge for the field, because doing work in communities is its own expertise,” Zarecor explained. Even though the residents in rural Iowa are the direct beneficiaries of the work from Zarecor’s team, the knowledge gap with respect to finding and using data makes those benefits inaccessible to some residents. Meanwhile, data scientists often lack the skills to convey their findings to an audience outside their academic circle. “As a field, data science, in my opinion, has not done a good job to educate necessarily well-rounded [data scientists].”
To overcome this bottleneck, Zarecor’s team works on creating dashboards that visualize the data and make the data more interpretable to the rural communities. Zarecor also encourages the statisticians on her team to talk to residents of the communities they study and ask what kind of data they would like to have. “When we ask what they want, it’s not because they know everything that’s available. We’re doing a mix of hearing from them what they want, and also guessing some things that they probably don’t know are out there that we can also give them in a usable form.”
Zarecor believes that similar types of highly collaborative and interdisciplinary research would benefit the entire research community, and those collaborations start with abandoning assumptions of different fields.
She gives an example in the discipline of architecture, where architects would assume themselves to be capable of doing graphic design or planning. Many don’t realize that those tasks are outside of their expertise even though these fields are seemingly adjacent. “And I would transfer that over to data scientists who know that data science is a synthetic and integrative discipline. [. . .] It doesn’t mean, though, that there are not all of these soft skills, all of this other communication, and people-related aspects of the data science work that you can handle without help.”
Therefore, Zarecor suggests that data scientists should work in conjunction with domain experts to make their research more relatable to a broader audience. Team members also need to respect the importance and specificity of other kinds of expertise beyond the technical or data-driven parts of a project. When a team successfully works this way, “the data science gets improved and amplified and becomes more useful. If you actually think horizontally on the project, you know that there’s not a pyramid, but that you are a team that’s working across the group [of collaborators]. This would be a much healthier way of [working with] data and for data scientists to interact with people.”
In this regard, Zarecor noted that the Midwest Big Data Innovation Hub, as a highly integrated and inclusive organization, has the potential to cultivate different layers of collaboration across various disciplines. “But it does require the data scientists who were the first audience, or the more explicit audience [for the Hub], to be willing to open up.”
Get Involved
New community-building activities in the Smart & Resilient Communities priority area of the Midwest Big Data Innovation Hub are beginning in spring 2022. Contact the Hub if you’re interested in participating, or are aware of other people or projects we should profile here. The MBDH has a variety of ways to get involved with our community and activities
The Midwest Big Data Innovation Hub is an NSF-funded partnership of the University of Illinois at Urbana-Champaign, Indiana University, Iowa State University, the University of Michigan, the University of Minnesota, and the University of North Dakota, and is focused on developing collaborations in the 12-state Midwest region. Learn more about the national NSF Big Data Hubs community.