By Jas Mehta
For decades, a fundamental question in biology remained largely unanswered: how do proteins fold? Proteins, large, complex molecules, play crucial roles in virtually every biological process within our cells. These building blocks, the workhorses of our cells, contort their amino acid chains into intricate 3D shapes that dictate their function. Unveiling these structures has been a slow and expensive endeavor, hindering progress in medicine, drug discovery, and our understanding of life itself.
Researchers have grappled with the challenge of deciphering protein structures using methods such as X-ray crystallography and computational modeling. However, these approaches often fell short in terms of accuracy and efficiency. Scientists and software developers using artificial intelligence (AI) concepts are creating powerful new tools to address this challenge. One example, called AlphaFold, was developed by the DeepMind subsidiary of Google’s parent company, Alphabet. AlphaFold represents a paradigm shift in protein structure prediction, building upon decades of research engaging with the intricate puzzle of protein folding, and leveraging the power of deep learning to achieve near-atomic accuracy in predicting 3D protein structures from amino acid sequences. (See the image below for an example of how researchers are computing protein structure from amino acid sequence data.)
Computing protein structure from amino acid sequence
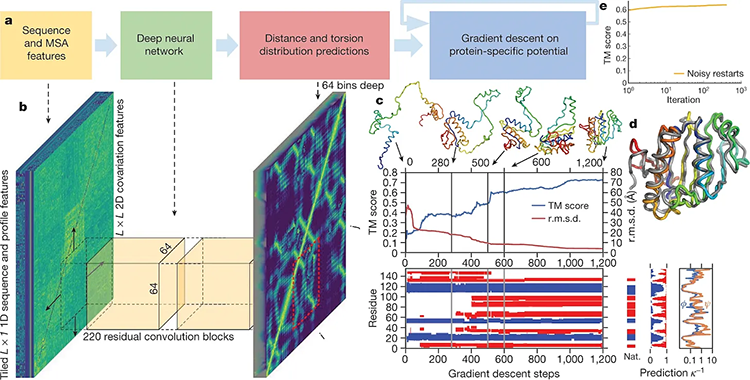
This breakthrough has not only streamlined the process, reducing prediction times from months to minutes, but has also opened new avenues for drug discovery and biomedical research, promising to revolutionize our understanding of proteins and their functions within cells. This represents a monumental leap compared to traditional methods like X-ray crystallography, which can take months or even years. This breakthrough not only accelerates research cycles and slashes costs but also holds profound implications for fields ranging from medicine to materials science.
In the 14th Critical Assessment of Protein Structure Prediction (CASP), a biennial competition, AlphaFold achieved a staggering feat. It matched or surpassed the accuracy of experimental methods for a whopping 90% of proteins, showcasing the immense power of deep learning for this complex task. Historically, determining a protein structure could cost upwards of $100,000 and take months. AlphaFold slashes this time to minutes, with a projected cost per prediction of mere cents. This translates to significant cost savings and faster research cycles.
Designing drugs often hinges on knowing a protein’s structure. AlphaFold’s speed and accuracy streamline this process. A recent study used AlphaFold to identify a potential drug target for a baffling neurodegenerative disease, a process that would have taken significantly longer using traditional methods. Moving beyond snapshots, the next frontier is understanding how proteins fold, move, and interact within the cell. This will provide invaluable insights into cellular processes and protein function. Deep learning thrives on data. Integrating protein interaction databases, cellular environment data, and real-time folding kinetics will further enhance the accuracy and applicability of protein structure prediction. Open-source platforms like AlphaFold are making these powerful tools accessible to researchers worldwide. This fosters collaboration and accelerates scientific progress across disciplines.
The success of AlphaFold stands as a testament to the indispensable role played by the Protein Data Bank (PDB), a vast repository housing experimentally determined protein structures. Mr. Darnell Granberry, a distinguished machine-learning (ML) engineer at the New York Structural Biology Center, sheds light on the critical importance of open data in driving groundbreaking advancements in protein research. “The PDB contains nearly all of the protein structures that have been experimentally determined, and the fact that it’s open source is a major enabler of AlphaFold and other protein ML models,” remarks Mr. Granberry. “If we didn’t have it, I think we’d likely have been limited to in-house models developed at pharma/biologics companies on proprietary data.”
His insights offer a nuanced understanding of the symbiotic relationship between computational methods and protein research, emphasizing the transformative impact of accessible data on scientific innovation. Furthermore, Mr. Granberry eloquently articulates a foundational principle of biology, stating, “There’s that central dogma of biology: DNA to RNA, RNA to protein, protein to function. So basically, anything that you’re interested in, basically in any living thing, is going to be rooted in some sort of protein or complex of proteins, or collection of them that interact with each other.”
In his words, we discern a profound appreciation for the pivotal role played by proteins in shaping the essence of life itself, underscoring the fundamental importance of unraveling their structures and functions in driving progress across diverse realms of scientific inquiry.
In a recently published study, researchers used AlphaFold to predict the structure of a protein implicated in amyotrophic lateral sclerosis (ALS), a debilitating neurodegenerative disease. The predicted structure revealed a never-before-seen binding site, paving the way for the design of drugs that could potentially slow or halt disease progression. This exemplifies AlphaFold’s potential to revolutionize drug discovery, particularly for complex and previously untreatable diseases.
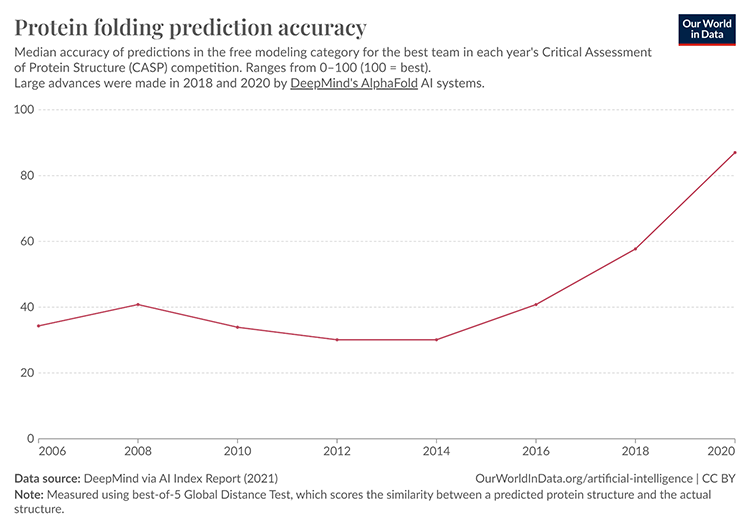
The chart above depicts the median accuracy of protein-folding predictions in the free-modeling category of the CASP competition over the years. As you can see, there was a significant jump in accuracy in 2018 and 2020, coinciding with the introduction of DeepMind’s AlphaFold systems. This dramatic improvement highlights the transformative power of deep learning in protein-folding prediction.
Deep learning has irrevocably transformed protein-folding prediction. As we delve deeper into protein dynamics and leverage the power of big data, the potential applications are truly boundless. From developing new medicines and biomaterials to a fundamental understanding of how life works at the molecular level, AlphaFold and its successors promise to usher in a new era of biological discovery.
Get Involved
Contact the Midwest Big Data Innovation Hub if you’re aware of other people or projects we should profile here, or to participate in any of our community-led Priority Areas. The MBDH has a variety of ways to get involved with our community and activities. The Midwest Big Data Innovation Hub is an NSF-funded partnership of the University of Illinois at Urbana-Champaign, Indiana University, Iowa State University, the University of Michigan, the University of Minnesota, and the University of North Dakota, and is focused on developing collaborations in the 12-state Midwest region. Learn more about the national NSF Big Data Hubs community.